Chapter 2 Nonlinear Principal Components Analysis. Correspondence Analysis in the Social Sciences.
Pdf Principal Components Analysis With Nonlinear Optimal Scaling Transformations For Ordinal And Nominal Data Semantic Scholar
Journal of Personality Assessment.
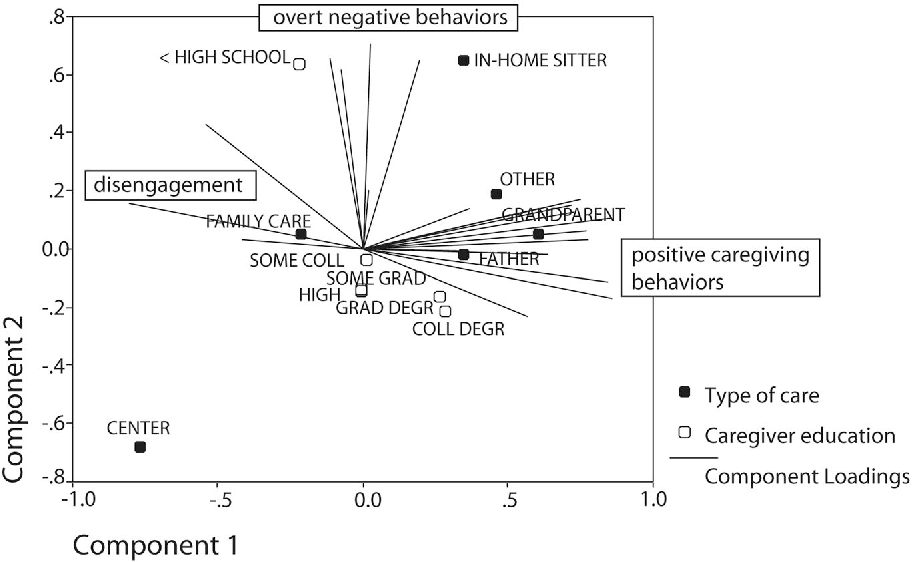
. Factor analysis and principal components analysis. The goal of this paper is to dispel the magic behind this black box. You can create one manually by copying the eigenvalues out of the Model Summary table in the output or if you will need to create a lot of scree plots you can use the SPSS Output Management System OMS to automate pulling the values out of the table and creating the plot.
Request PDF Nonlinear principal components analysis with CATPCA. Linting and Van der Kooij 2012. Without making any assumptions about the measurement levels of the variables.
This method is the nonlinear equivalent of stan- dard PCA and reduces the observed variables to a number of uncorrelated principal components. Nonlinear principal components analysis with catpca. Nonlinear principal components analysis with CATPCA.
The nonlinear categorical principal component analysis method CATPCA is an extended version of this method for categorical data ie ordinal and nominal data. This article is set up as a tutorial for nonlinear principal components analysis NLPCA systematically guiding the reader through the process of analyzing actual data on. This article is set up as a tutorial for nonlinear principal components analysis NLPCA systematically guiding the reader.
This article is set up as a tutorial for nonlinear principal components analysis NLPCA systematically guiding the reader through the process of analyzing actual data on personality assessment by the Rorschach Inkblot Test. The goal of principal components analysis is to reduce an original set of variables into a smaller set of uncorrelated components that represent most of the information found in the original variables. The most important advantages of nonlinear over linear PCA are that it incorporates nominal and ordinal variables.
Institute for Nonlinear Science University of California San Diego La Jolla CA 92093-0402 Dated. The results of the factor analysis were used to weight the new SCI. Ibm spss categories 19.
The authors provide a didactic treatment of nonlinear categorical principal components analysis PCA. Suppose that some CATPCA-analyzed ordinal or dichotomous variable A is higher value for those who agree in that question asked. Exploring the distribution of variables and their nonlinear relationships.
Return to the SPSS Short Course. A tutorial This article is set up as a tutorial for nonlinear principal components analysis NLPCA systematically guiding the. Introduction and Application This chapter provides a didactic treatment of nonlinear categorical principal components analysis PCA.
NLPCA is a more flexible alternative to linear PCA that can handle the analysis of possibly nonlinearly related variables with different types of. One of the most commonly used methods to reduce the dimension of data and reveal hidden patterns is the principal component analysis PCA. Begingroup You can save principal scores from the CATPCA procedure as new variables to your dataset and then analyse them as any other variables.
Nonlinear principal components analysis. Consequently the optimally scaled variables were used as input for factor analysis with principal component extraction. This method is the nonlinear equivalent of standard PCA and reduces the observed variables to a number of uncorrelated principal components.
Relevant clinical information can influence the diagnostic probability and reporting of ultrasound findings. Categorical principal components analysis CATPCA is appropriate for data reduction when variables are categorical eg. Then if A is positively loaded by the component then those who have a higher component.
Nonlinear principal components analysis with CATPCA. Nonlinear principal components analysis. The authors provide a didactic treatment of nonlinear categorical principal components analysis PCA.
Nonlinear Principal Component Analysis NLPCA was conducted on the categorical data to reduce the observed variables to uncorrelated principal components. Nonlinear principal components analysis with CATPCA. This article is set up as a tutorial for nonlinear principal components analysis NLPCA systematically guiding the reader through the process of analyzing actual data on personality assessment by the Rorschach Inkblot Test.
Please participate in the DSA Client Feedback Survey. Version 2 Principal component analysis PCA is a mainstay of modern data analysis - a black box that is widely used but poorly understood. This method is the nonlinear equivalent of standard PCA and reduces the observed variables to a number of uncorrelated principal components.
CATPCA does not produce a scree plot. The subscales of job. This article is set up as a tutorial for nonlinear principal components analysis NLPCA systematically guiding the reader through the process of analyzing actual data on personality assessment by the Rorschach Inkblot Test.
Up to 10 cash back To avoid this limitation categorical principal component analysis CATPCA or nonlinear principal component analysis NLPCA has been introduced as an alternative in dealing with nominal and ordinal data Linting et al. NLPCA is a more flexible alternative to linear PCA that can handle the analysis of possibly. Pca can principal component analysis be applied to datasets.
CATPCA was applied to the training set and resulted in a two-component solution. Merge categories based on transformation plots. Recent Developments and Applications M.
Ordinal and the researcher is concerned with identifying the. Categorical principal components analysis is also known by the acronym CATPCA for categorical principal components analysis. Categorical Principal Components Analysis CATPCA with Optimal Scaling.
Nonlinear principal components analysis with catpca. NLPCA is a more flexible alternative to linear PCA that can handle the ana. The job satisfaction items were measured at an ordinal scaling level and analyzed by Categorical Principal Components analysis CATPCA using monotonic spline transformations.
The sample was randomly divided into a training set and a test set.
Pdf Principal Components Analysis With Nonlinear Optimal Scaling Transformations For Ordinal And Nominal Data Semantic Scholar
Pdf Principal Components Analysis With Nonlinear Optimal Scaling Transformations For Ordinal And Nominal Data Semantic Scholar
Pdf Nonlinear Principal Components Analysis Introduction And Application Semantic Scholar
Pdf Nonlinear Principal Components Analysis Introduction And Application Semantic Scholar
Pdf Nonlinear Principal Components Analysis Introduction And Application Semantic Scholar
Pdf Nonlinear Principal Components Analysis Introduction And Application Semantic Scholar
Pdf Nonlinear Principal Components Analysis Introduction And Application Semantic Scholar
0 comments
Post a Comment